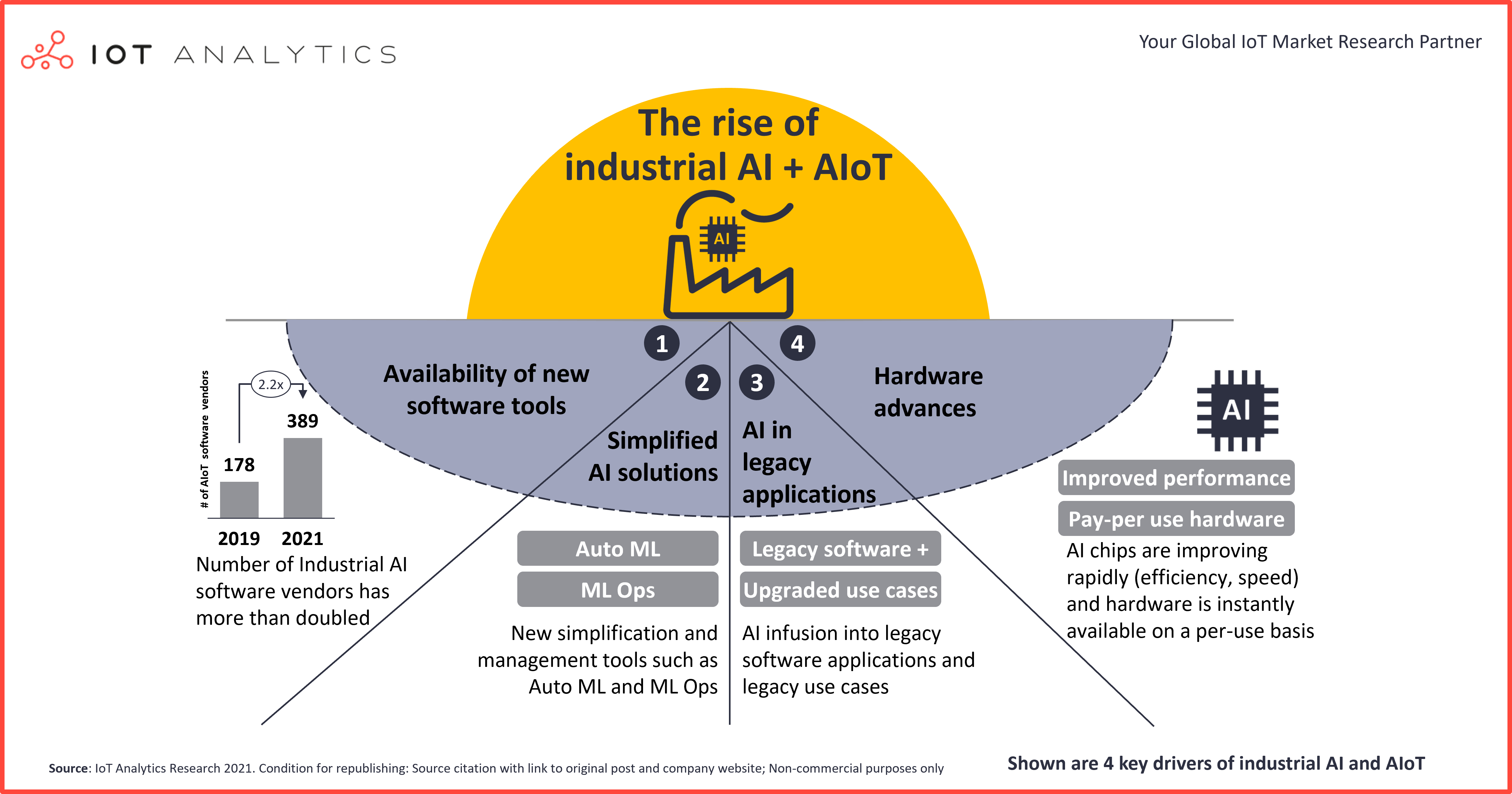
In short
- Two new IoT Analytics research reports on the topics of industrial artificial intelligence (AI) and the Artificial Intelligence of Things (AIoT) estimate that the AIoT market will reach $102.2 billion by 2026. (Report 1, Report 2)
- Four trends are driving the technology: (1) the availability of new software tools, (2) the development of simplified AI solutions, (3) the infusion of AI into legacy applications, and (4) advances in AI hardware.
Why it matters
- Manufacturing software and hardware providers have the opportunity to infuse AI-based features into existing offerings.
- Manufacturers who adopt these solutions wisely are witnessing increased efficiency, higher product quality, and smoother processes.
The adoption of AIoT in manufacturing is growing.
The AI adoption rate in industrial settings has increased from 19% to 31% in slightly more than two years, according to data from the recently released 252-page Industrial AI and AIoT Market Report 2021–2026. On top of the 31% of respondents that have fully or partially rolled out AI technology in their operations, an additional 39% are currently testing or piloting the technology. Increased AI adoption can be witnessed across the board but is especially strong in the energy vertical and in process industries, such as oil and gas or chemicals. The combination of high-value assets, large volumes of operational data, and processes that rely on hundreds of parameters contributes to the strong adoption in these industries. Common industrial AI applications include maintenance (e.g., predictive maintenance [PdM]), predictive quality control, the use of machine vision for fault detection, AI-optimized inventory management, and AI-based production planning and optimization.
AI is becoming a key technology for manufacturers and energy companies globally, and IoT Analytics forecasts the market for industrial AI solutions will exhibit a strong post-pandemic compound annual growth rate (CAGR) of 35%, with the market reaching $102.17 billion by 2026.
| What is industrial AI and industrial AIoT?
Industrial AI = AI related to an industrial enterprise’s physical operations and systems. AI-driven systems can automate and reinvent fundamental industrial processes, including product development/manufacturing and supply chain/field operations.
Industrial AIoT = A subset of industrial AI that refers to AI performed on IoT-type data sources in industrial enterprises.
It is increasingly obvious that true AI (not just simple statistics) will become pervasive in many manufacturing operations and related applications in the coming years.
According to our research, four key components are driving the widespread adoption of AI within industrial settings.
Driver 1: Software tools are increasingly available for industrial AIoT.
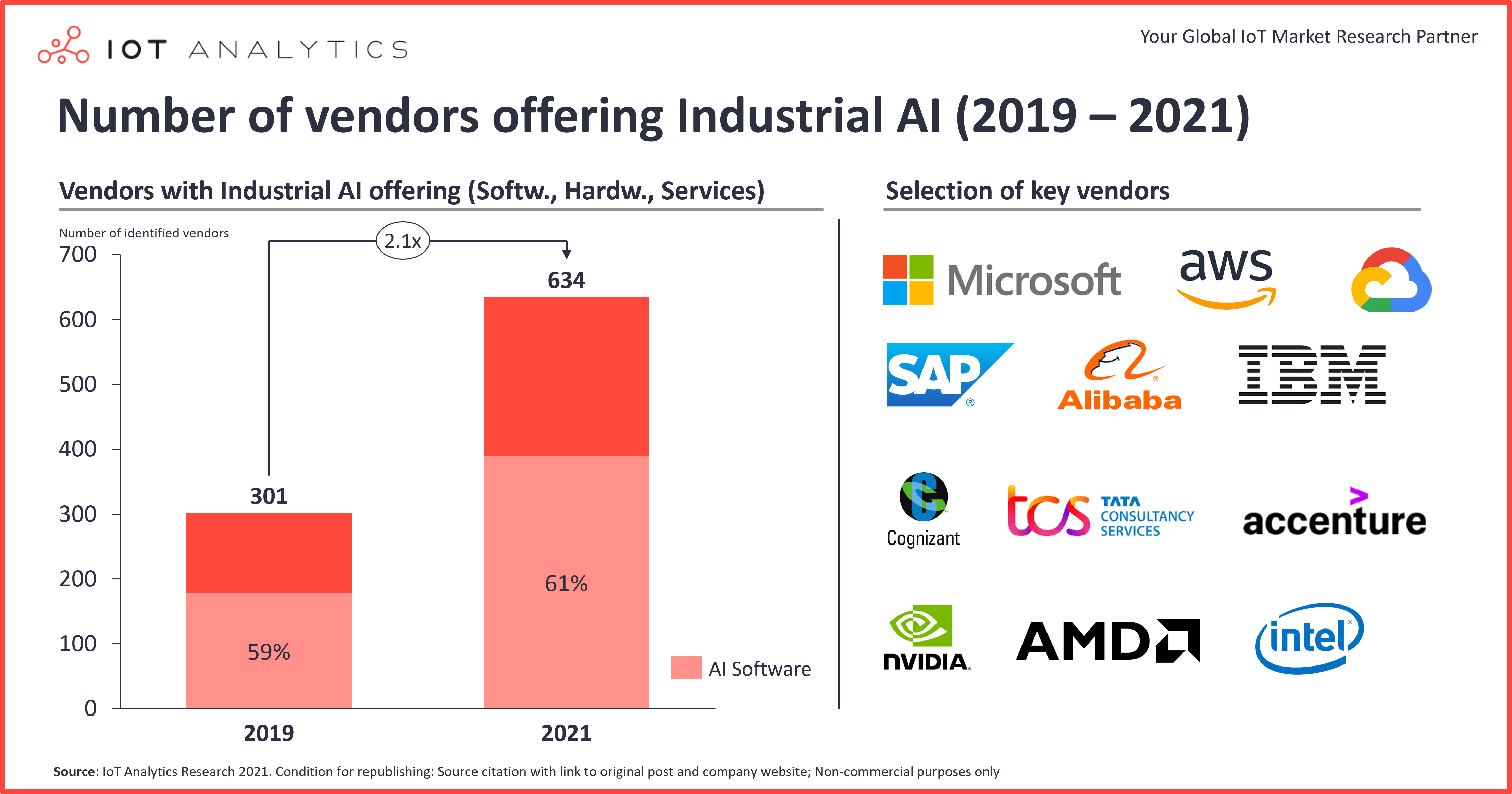
Nearly 400 vendors offer AIoT software. The number of software vendors joining the industrial AI market has considerably increased in the last two years. During the research, IoT Analytics identified 634 vendors offering AI technology for manufacturers/industrial clients. Of these firms, 389 (or 61.4%) offer AI software.
New AI software platforms focus on industrial settings. In addition to software scale-ups, such as Uptake, Braincube, or C3 AI, an increasing number of operational technology (OT) vendors are offering dedicated AI software platforms. In 2019, when IoT Analytics started covering the industrial AI space, there were very few dedicated AI software offerings from these companies. Since then, many OT vendors have entered the AI market by developing and offering AI software solutions in the form of AI platforms for the factory floor. Examples include ABB’s Genix Industrial Analytics and AI Suite, Rockwell Automation’s FactoryTalk Innovation Suite, Schneider Electric’s Autonomous Production Advisor platform and specific recent add-ons such as TangentWorks’ InstantML to Siemens’ MindSphere platform. Several of these platforms are aimed at a wide range of use cases. For example, ABB’s Genix platform offers advanced analytics with pre-built applications and services for operational performance management, asset integrity, sustainability, and supply chain efficiency.
The newly released 172-page Industrial AI Software Platform Vendor Landscape Report 2021 takes a deep-dive into 12 of these vendors.
Hyperscalers are extending their offerings to the shopfloor. The increased availability of AI software tools is also driven by the development of new use-case-specific software tools from hyperscalers, including AWS, Microsoft, and Google. For instance, in December 2020, AWS released Amazon SageMaker JumpStart, a capability of Amazon SageMaker that offers a set of pre-built and customizable solutions for the most common industrial use cases (e.g., PdM, computer vision, and autonomous driving) that can be deployed with just a few clicks.
Use case-specific software solutions are driving increased availability. Use-case-specific software suites, such as those focused on Predictive Maintenance, are becoming more common. IoT Analytics observed that the number of providers using AI-based PdM software solutions rose to 73 in early 2021 due to an increase in the variety of data sources and the use of pre-trained models, along with the widespread adoption of data augmentation techniques (see the Predictive Maintenance Market Report 2021–2026 for more details).
Driver 2: The development and maintenance of AI solutions is being simplified.
Automated machine learning (AutoML) is becoming a standard offering. As machine learning (ML)-related tasks are complex, the rapid growth of ML applications has created a demand for off-the-shelf ML methods that can be used without expert knowledge. The resulting research area, which targets the progressive automation of ML, is called AutoML. Various companies are leveraging the technology as part of their AI offerings to help clients develop ML models and realize industrial use cases faster. For instance, in November 2020, SKF announced an AutoML-based offering that combines machine process data with vibration and temperature data to reduce costs and enable new business models for its customers. Similarly, early this year, C3 AI announced the availability of AutoML in C3 AI Ex Machina.
“Building an ML model manually requires extensive domain expertise and significant time spent on trial and error. Selecting training features, [a] model type, and the specific configuration of that model that optimizes accuracy are difficult problems even for expert data scientists. Automated ML eliminates this tedious experimentation process, saving time and trouble for both business experts and data scientists.”
– Matt Connor, senior product manager focused on C3 AI Ex Machina
Machine learning operations (ML Ops) eases model management and maintenance. The new discipline of ML Ops aims to streamline AI model maintenance within manufacturing settings. The performance of AI models often degrades over time, as it is affected by several factors within the factory (e.g., changes in data distribution and quality standards). Therefore, model maintenance and ML Ops have become necessary to meet the high quality demands of industrial settings (for example, a model performing below 99% accuracy could fail to identify behaviors that jeopardize workers’ safety). Many startups have joined the field of ML Ops in recent years, including DataRobot, Grid.AI, Pinecone/Zilliz, Seldon, and Weights & Biases. Established companies have added ML Ops capabilities to their existing AI software offerings, including Microsoft, which introduced Data Drift Detection in Azure ML Studio. This new feature enables users to detect changes in the distribution of input data that lead to model performance degradation.
Driver 3: AI is being infused into existing applications and use cases.
Legacy software providers are adding AI features. In addition to the existing big horizontal AI software tools (e.g., MS Azure ML, AWS SageMaker, and Google Cloud Vertex AI), legacy software suites, such as computerized maintenance management systems (CMMSs), manufacturing execution systems (MESs), or enterprise resource planning (ERP), can now gain a significant boost through the infusion of AI capabilities. For instance, ERP provider Epicor Software is adding AI capabilities to its existing offering through its Epicor Virtual Assistant (EVA). The intelligent EVA agent is used to automate ERP processes, such as rescheduling manufacturing jobs or executing simple queries (e.g., getting specifics on product pricing or available part quantities).
Industrial use cases are being upgraded through the use of AIoT. Several industrial use cases are being enhanced through the addition of AI capabilities to existing hardware/software infrastructures. A vivid example is that of machine vision in quality control applications. Legacy machine vision systems process images through an integrated or discrete computer equipped with specialized software that evaluates predetermined parameters and thresholds (e.g., high contrast) to determine whether an object exhibits a defect. In many contexts (e.g., electronic assemblies with different wiring shapes), the number of false positives is very high. However, these systems are seeing a revival with AI. For instance, Cognex, an industrial machine vision provider, released a new deep learning tool (Vision Pro Deep Learning 2.0) in July 2021. The new tool integrates with legacy vision systems, enabling end users to combine deep learning with traditional vision tools in the same application for demanding medical and electronics contexts that require precise measurements of scratches, contamination, and other defects.
“As deep learning gains a foothold in the factory, we are seeing that many applications require more than just traditional vision or deep learning alone—they need both for the best solution.”
– Joerg Kuechen, senior vice president of Cognex vision products
Driver 4: Hardware for industrial AIoT is improving.
AI hardware has evolved since our last industrial AI report in 2019, and there are more dedicated offerings to support industrial AIoT use cases.
AI chips are improving rapidly. Embedded hardware AI chips have witnessed rapid development, and multiple options are available to support the development and deployment of AI models. Examples include NVIDIA’s latest graphics processing units (GPUs), A30 and A10, which were introduced in March 2021 and are suitable for AI use cases, such as recommender systems and computer vision systems. Another example is Google’s fourth generation of tensor processing units (TPUs), which are powerful application-specific integrated circuits (ASICs) that can achieve up to 1,000x efficiency and speed in both model development and deployment for specific AI workloads (e.g., object detection, image classification, and recommendation benchmarks).
The use of dedicated AI hardware reduces model calculation time from days to minutes and has proved to be a game changer in many instances.
Powerful AI hardware is available instantly via pay-per-use models. Hyperscalers are constantly upgrading their servers to make computing resources available in the cloud so that end users can realize industrial AI applications. For instance, in November 2021, AWS announced the general availability of its latest GPU-based instances, Amazon EC2 G5, which are powered by NVIDIA A10G Tensor Core GPUs for use in a wide range of ML applications, including computer vison and recommender engines. Inspection system provider Nanotronics, for example, leverages Amazon EC2 instances for its AI-based quality control solutions to expedite processing jobs and achieve more accurate detection rates in the manufacturing of microchips and nanotubes.
Conclusion and outlook
AI is coming to the factory, and it will be pervasive in new applications, such as AI-based PdM, and as an augmentation to existing software and use cases. Large enterprises are rolling out several AI use cases and reporting successes, with strong ROI for most projects. Altogether, the rise of the cloud, IoT platforms, and powerful AI chips provides a platform for a new generation of software and optimization.
What should you do now?
For manufacturing software and hardware providers alike, it is time to holistically evaluate the use of AI and ML to augment your existing product portfolio (e.g., through the introduction of smart assistants, simulation tools, or predictive analytics). This AI augmentation could be transformative (as the introduction of AI-based machine vision has been in quality inspection).
For manufacturers, it is time to refine your existing Industry 4.0 or industrial IoT strategy with a clear industrial AI vision and decide how AI technology should be acquired (e.g., hardware sourced from a cloud provider vs. in-house dedicated AI hardware or partnership with an AI software startup vs. building an internal AI team). Manufacturers also need to find answers to crucial questions: Who are we willing to share data with to improve operations? How do we achieve acceptance of AI on the shopfloor? And how do we enable the workforce to use AI-based applications in their everyday tasks?
Let our research guide you. Our 2 recent market reports were developed to provide both vendors and users of Industrial AI technology with an immediate overview of the market, the key use cases, the technology, the adopter’s perspective and more – all with numerous examples and case studies.
More information and further reading
Are you interested in learning more about Industrial AI and AIoT?
IoT Analytics has released two publications on the topic as part of our ongoing coverage of IoT Platforms and Software (IoT Software Workstream).
1. Industrial AI and AIoT Market Report
The Industrial AI and AIoT Market Report 2021-2026 is a comprehensive 252-page report assessing the Industrial AI and AIoT market. It also includes a comprehensive summary of the results of two industrial AI end user surveys, a technology overview, a vendor landscape with ~630 firms, an in-depth analysis of industrial AI business models, detailed use case analysis and 6 case studies, as well as a discussion of eleven current trends and two challenges. It is part of IoT Analytics’ ongoing coverage of IoT Software/Platforms (IoT Software Workstream).
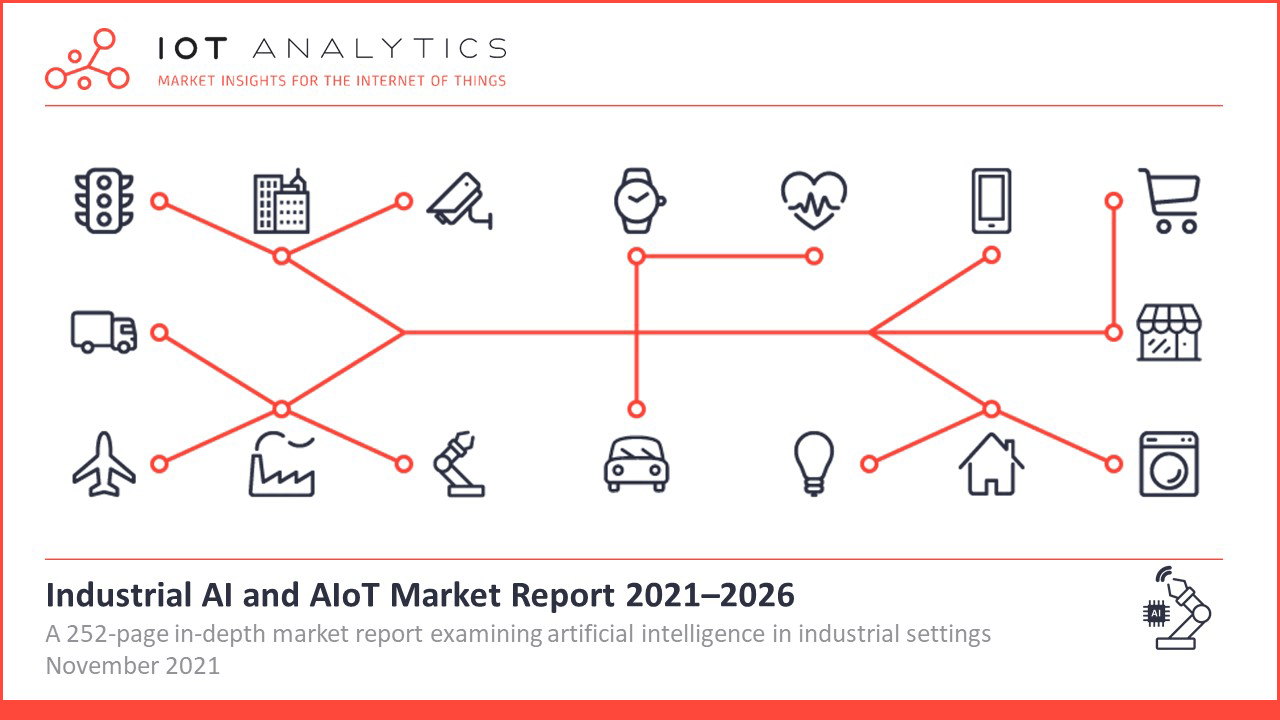
This report provides answers to the following questions (among others):
- How is Industrial AI defined?
- What are the major differences between industrial and non-industrial AI solutions?
- How has the industrial AI and AIoT market evolved since 2018?
- How big is the industrial AI and AIoT market and how fast is it growing across various dimensions (by offering type, region, industry, use case)?
- How many start-ups have joined the market and what are their funding details?
- Which companies are leading the market (by offering type, including market shares)?
And more …
Sample:
The sample of the report gives you a holistic overview of the available analysis (outline, key slides). The sample also provides additional context on the topic and describes the methodology of the analysis. You can download the sample here:
2. Industrial AI Software Platform Vendor Landscape Report 2021
The Industrial AI Software Platform Vendor Landscape Report 2021 includes an in-depth analysis of 12 selected OT software vendors offering industrial AI solutions, including information on end users’ usage and satisfaction feedback for those selected 12 vendors and several others within the industrial AI market. The information presented in this report is based on extensive secondary research as well as interviews and surveys of vendors and end-users conducted between April and November 2021.
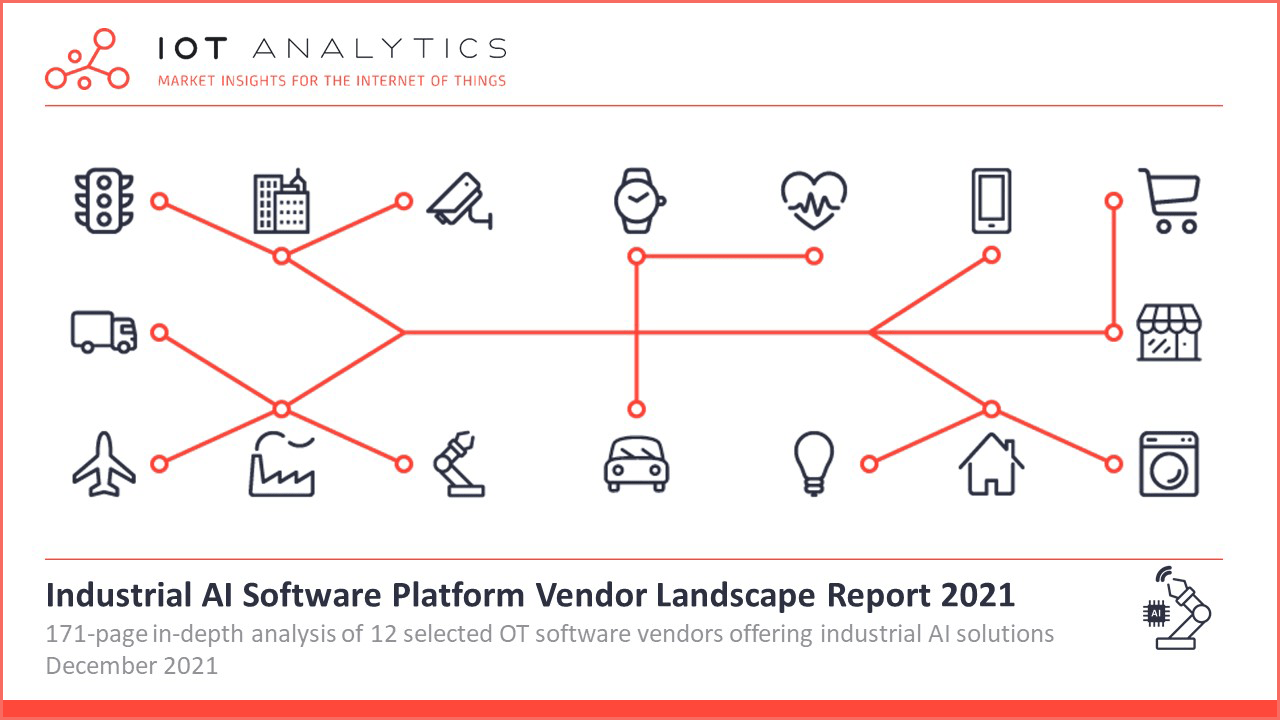
This report provides answers to the following questions (among others):
- What is the industrial AI strategy of 12 selected Industrial AI software platform vendors?
- What are the components of those software vendors’ industrial AI solutions?
- Which are the technology partners of those software vendors in the industrial AI market?
- How do those software vendors compare to each other in terms of usage and satisfaction levels for their AI platform and solutions?
- How do those AI platform vendors sell their AI offerings?
And more…
Sample:
The sample of the report gives you a holistic overview of the available analysis (outline, key slides). The sample also provides additional context on the topic and describes the methodology of the analysis. You can download the sample here:
Related articles
You may also be interested in the following recent articles:
- 6 IoT adoption trends for 2022
- Predictive Maintenance Market: The Evolution from Niche Topic to High ROI Application
Are you interested in continued IoT coverage and updates?
Subscribe to our newsletter and follow us on LinkedIn and Twitter to stay up-to-date on the latest trends shaping the IoT markets. For complete enterprise IoT coverage with access to all of IoT Analytics’ paid content & reports including dedicated analyst time check out Enterprise subscription.