Research findings from an analysis of 100 digital twin projects
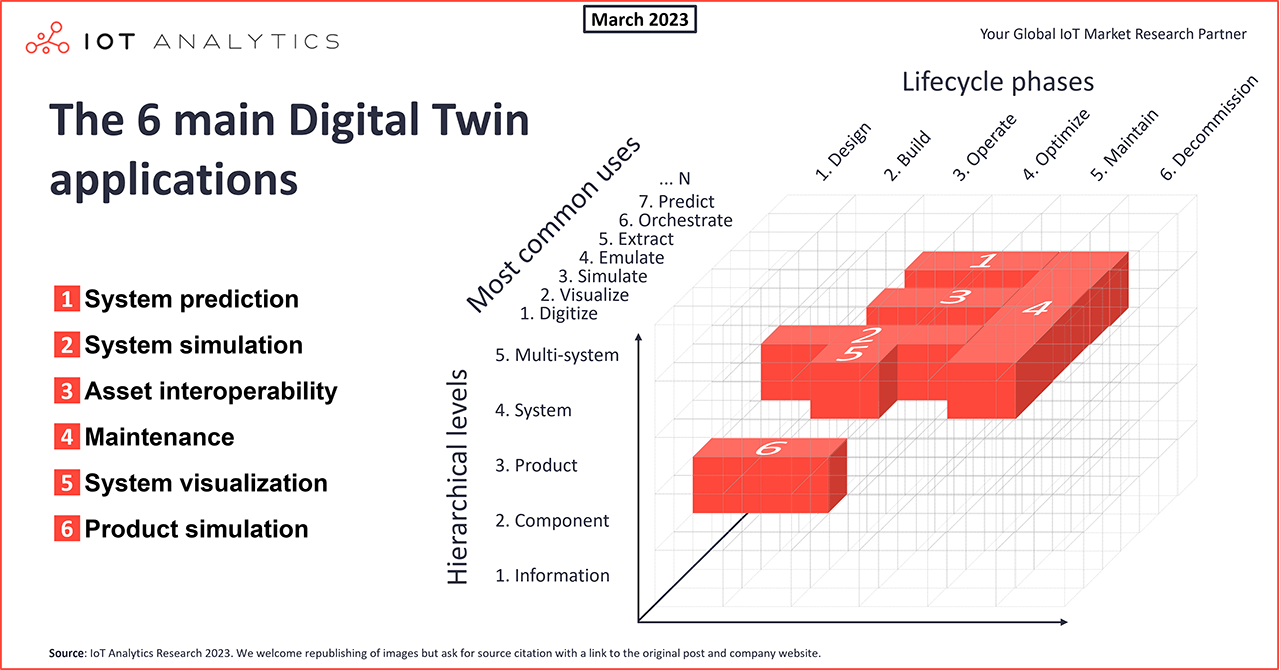
In short
- The market for digital twins expanded by 71% between 2020 and 2022. 63% of manufacturers are currently developing a digital twin or have plans to develop a digital twin.
- The latest Digital Twin Market Report 2023–2027 highlights the six main digital twin applications today: system prediction, system simulation, asset interoperability, maintenance, system visualization, and product simulation.
Why does it matter?
- Many digital transformation initiatives involve the creation of digital replicas of physical assets (digital twins).
- Understanding the nuances of different digital twins and activity hotspots helps vendors focus their efforts and end users pick the most value-generating initiatives.
Click on the button to load the content from .
Digital Twins have become a key topic for Industry 4.0
The market for standalone digital twin software grew 71% between 2020 and 2022, according to the recently published Digital Twin Market Report 2023–2027. Survey results, also part of this research, indicate strong adoption. For example, 29% of manufacturing companies globally have fully implemented or are implementing a digital twin strategy for a portion of their operational assets, and a further 63% are currently developing or have already developed their digital twin strategy.
Although the roots of digital twins go back to NASA’s Apollo program in 1970, the concept of creating digital replicas of physical assets and visualizing/simulating/predicting in a virtual world is extremely suitable for companies that are trying to make Industry 4.0 a reality or are aiming toward future industrial metaverse projects.
Make no mistake: While the definition of a digital twin may be straightforward, its applications are numerous. In 2020, we published our first market research on the topic and showcased that there may, in fact, be 200 or more different types of digital twins.
The feedback we received from you was that classification helps to ensure apple-to-apple digital twin comparisons, but questions remain about the hotspots of activity. Therefore, as part of our new 233-page Digital Twin Market Report 2023-2027, we classified 100 real digital twin projects along the three dimensions and found six main areas of activity. These six digital twin application hotspots cover two thirds of all digital twin projects we analyzed.
Classifying Digital Twins
IoT Analytics defines a digital twin as a virtual model replicating the behavior of an existing or a potential real-world asset, system, or multiple systems.
There are three main dimensions describing the concept of digital twins. Each axis of the cuboid represents one dimension of the digital twin:
- Life cycle phase: The X-axis represents the six life cycle phases a digital twin is used for, from design to decommissioning.
- Hierarchical levels: The Y-axis represents the five hierarchical levels a digital twin represents, from information to multi-system.
- Use/purpose of implementation: The Z-axis represents the seven most common uses for digital twins, such as simulation and prediction.
There are 210 potential different digital twin combinations (5 x 6 x 7 = 210), although our research indicates that many digital twin initiatives cater to more than one combination.
The six most common Digital Twin applications
As part of the research, IoT Analytics looked at 100 digital twin case studies and classified each project into the digital twin cuboid. The result was that six clusters of digital twin activity stand out. We call them digital twin applications:
Digital twin application | Description | % of projects | |
---|---|---|---|
1. | Twins for system prediction | A digital twin geared toward predicting complex systems | 30% |
2. | Twins for system simulation | A digital twin geared toward simulating complex system behavior | 28% |
3. | Twins for asset interoperability | A digital twin geared toward common data formats and streamlined data extraction in complex systems | 24% |
4. | Twins for maintenance | A digital twin geared toward assisting with maintenance-related use cases | 21% |
5. | Twins for system visualization | A digital twin geared toward visualizing a complex system (e.g., in 3D) | 20% |
6. | Twins for product simulation | A digital twin geared toward simulating the behavior of (future) products (mostly during the design phase) | 9% |
Digital Twin application 1: System prediction
30% of the analyzed digital twin projects are considered digital twins for system prediction, where the digital twins are used for predicting entire systems (such as a part or the entirety of a factory, building, wind farm, or city) during its “operate” or “optimize” life cycle phase.
Such digital twins focus on predicting the behavior and future state of a physical system using current data and relevant records of the operational history. At the core of predictive digital twins are predictive models that are used to predict future outcomes.
System prediction Digital Twin project example:
Korean wind farm operator Doosan Enerbility deployed a digital twin to help predict power output, thus aiding the operations of their wind farms. The digital twins incorporate data from IoT sensors on wind turbines along with weather data and a model of expected power output. The digital twin serves two main purposes: a.) Acting as a performance watchdog where a physics-based model of the wind turbines calculates theoretical output, taking into consideration the current weather conditions, comparing the theoretical output with the actual output, and flagging any anomalies. b.) Acting as an output prediction tool for improved planning that predicts future power generation based on forecasted weather data.
The digital twin-based power output predictions assisted Doosan in improving wind farm revenues by increasing the output commitments the company made to South Korean energy grid operators while avoiding fines for failing to meet those commitments.
For more information, see Doosan Wind Farm Digital Twin: Visualizing IoT and Machine Learning
Digital Twin application 2: System simulation
28% of the analyzed digital twin projects are considered digital twins for system simulation, where a system was being simulated either during its “build,” “operate,” or “optimize” life cycle phases.
Simulating entire (complex) systems allows engineers to test “what-if” scenarios in a large setting with many more interlinked variables playing a role than in product-level simulations. Examples include factory simulations prior to opening up or making significant changes, rail network simulation, or traffic simulation. One of the key benefits of such system simulations is to reduce costs by testing the types of assets used, key operational parameters, and other important system variables prior to making the changes.
System simulation Digital Twin project example:
Train manufacturer Siemens Mobility needed to provide 170 new high-speed trains to German train operator Deutsche Bahn AG. For these trains, Siemens developed a new car-based train control architecture that had not been deployed before. To reduce development costs, Siemens developed a digital twin that would simulate the entire train’s functionality of 40 different subsystems. The main feature of the solution was a functional simulation that used a representation of the electrical schema of the train in the simulated environment. Siemens estimates that the digital twin-based simulation reduced cost by $1 million to $8 million.
For more information, see Reducing Risk and Cost with Virtual High-Speed and Commuter Train Test
Digital Twin application 3: Asset interoperability
24% of the analyzed digital twin projects are considered digital twins for asset interoperability, where digital twins streamline common data formats and allow for standardized data in/output during the “operate” or “optimize” life cycle phase.
Asset interoperability twins allow for real-time extraction of data from assets along various dimensions, including asset features, characteristics, properties, statuses, parameters, measurement data, and capabilities. Although asset interoperability is an underlying goal of any digital twin project, the main idea when implementing a specific asset interoperability twin is to develop a standardized way to address assets and the ability to promptly integrate new assets to the overall system. Many of the specific asset interoperability twin initiatives align on interoperability frameworks provided by major industry consortia, such as the Digital Twin Consortium’s Interoperability framework or Platform Industrie 4.0/IDTA’s Asset Administration Shell.
Asset interoperability Digital Twin project example:
Formula One team Scuderia Ferrari built a digital twin to help integrate and analyze the various sensor data points coming from the vehicle and allowing diverse teams to collaborate on analyzing aerodynamics, power, vehicle dynamics, and race engineering.
The ability to have all data sources streamlined into a digital twin helps the team make faster and more data-driven decisions.
For more information, see Palantir Technologies + Scuderia Ferrari Partnership Overview
Digital Twin application 4: Maintenance
21% of the analyzed digital twin projects are considered digital twins for maintenance, where the main purpose of the digital twin is to assist (a system) during the “maintain” phase of the life cycle, often involving some form of “prediction.”
Digital twins catering to the maintain life cycle phase are mostly geared toward assuring a system’s operational effectiveness, e.g., by assisting maintenance personnel during scheduled downtime or repair tasks by providing them with in-depth information about the physical asset or system. Maintenance digital twins also enable predictive maintenance use cases that aim to prevent asset failures altogether, thereby avoiding costly downtimes.
Maintenance Digital Twin project example:
German utility company E.ON embarked on a project that allowed the company to shift to a more preventive, predictive, and risk-based method of maintaining its assets. As part of this project, E.ON implemented a cloud-based digital twin that is used for better prediction of asset failures. The digital twin allows the company to evaluate failure modes per asset type and determine the remaining life of the equipment fleet.
For more information, see DNV Creates Digital Twin for E.ON
Digital Twin application 5: System visualization
20% of the analyzed digital twin projects are considered digital twins for system visualization, where the digital twin is used to visualize a system during its “operate” life cycle phase.
Among the most common types of visualizations used are 3D visual elements (often using CAD drawings) that help to create better transparency, e.g., about the current operating conditions of the system.
System visualization Digital Twin project example:
The national railway operator of Italy, Ferrovie dello Stato, built a digital twin of the rail infrastructure that included more than 10,000 miles of tracks, stations, tunnels, bridges, signals, switches, electrification hardware, and IT systems that coordinate everything. The digital twin sourced the data from sensors such as cameras and GPS receivers and employed advanced learning algorithms to create an interactive 3D replica of the rail system. ArcGIS was used for 3D visualization of geographical elements, creating a complete visual inventory of the railway infrastructure.
The resulting digital twin helps railway managers in the control room to visualize infrastructure such as bridges and stations remotely throughout the rail network. It also helps the local staff prevent safety hazards and boost on-time performance.
For more information, see How Digital Twin Technology Is Helping Build a Smart Railway System in Italy
Digital Twin application 6: Product simulation
9% of the analyzed digital twin projects are considered digital twins for product simulation, where a (future) product is being simulated during the “design” or “build” life cycle phase.
Digital twins play a key role for developing new and improved products. The key use case is to simulate different designs of a potential future product prior to building it, thereby eliminating the need to build costly prototypes and allowing for quick testing of thousands (in some cases millions) of product variances. Product simulation digital twins often reside in or are closely connected to computer-aided engineering (CAE) software and sometimes computer-aided design (CAD) software. Typical types of simulations include fluid dynamics, mechanical performance, or compatibility of electromagnetics.
Product simulation Digital Twin project example:
German packaging and bottling machine manufacturer Krones used a digital twin to test new product designs for one of its automated beverage-packaging system. The company wanted to incorporate a dynamic tripod robot into the product design. To do so, the friction and force of gravity on sliding packages across a moving conveyor belt and many other similar factors had to be tested. Krones developed a model for the tripod robot by importing geometry and inertia data from STEP files exported from CAD software. The team tested many scenarios, e.g., injecting faults such as extremely high levels of friction, during the simulations to analyze the system behavior during such an event. By modeling and simulating the tripod robot, Krones was able to increase the performance efficiency of the robot, product development time was shortened, and testing time was significantly reduced.
For more information, see Krones Develops Package-Handling Robot Digital Twin
Our take
The research shows that there is strong interest in digital twins, but not all digital twins are equal. Every digital twin project is distinct in terms of its sophistication, life cycle phase to which it caters, and use.
It is important to realize that there is not one best or one single use for digital twins. It really depends on the end goal. For some digital twin applications, simulation may be the key use (e.g., when wanting to test “what-if” scenarios); for others, emulation may be the key use (e.g., when checking for configuration errors or when training operators); and for others still, prediction is the key use (e.g., when estimating future performance).
We identified six clusters of digital twin applications that reflect two-thirds of all digital twin projects today. With regard to the most common use, 31% of the digital twin projects are focused on prediction at the system level.
What it means to companies looking to adopt Digital Twins?
It is important for organizations to look at digital twins as a means to achieve broader digital transformation. Although companies often start small, many end up scaling and integrating digital twin initiatives into larger digital threads. Companies should consider questions such as:
- What problems should our digital twin solve?
- Do we need more than one digital twin project?
- Should we look at the digital twin with the broader mindset of all 200+ potential combinations?
Our research also indicates that despite the success of digital twins, 72% of the organizations that embarked on a digital twin project reported facing a challenge with the cost of building digital twin solutions.
As with any uncertain technology initiative, adopters should also:
- Assess their readiness to adopt digital twins in terms of the existing technology stack and the in-house talent. Our research highlights that a number of “legacy” software tools such as CAD, CAE, and PLM—but also new tools such as IoT Platforms and the cloud—play an important role when developing digital twins.
- Quantify the benefits of the digital twin, and compare it with the opportunity cost of not implementing a digital twin for the respective use cases/proposed application.
- Design indicators that help measure progress. Certain digital twin projects take years before they materialize because ontology mapping, creating complex visualizations, or developing suitable prediction algorithms sometimes take longer than anticipated.
What it means to companies in the Digital Twin vendor ecosystem?
Digital twin vendors currently enjoy favorable market conditions, with digital twins being one of the highest growth areas in enterprise software right now.
Vendors, however, may want to consider the following three aspects:
- You may need six different digital twin go-to-market strategies. The six digital twin application areas we highlighted in this article are distinctly different, with inherently different customer needs. Application areas such as system prediction and system simulation are hotspots right now, and more customers are ready to adopt them; however, as a vendor, are you addressing each cluster correctly in your go-to-market strategy?
- Partnerships are important. Vendors need to keep an eye on fine-tuning the existing digital twin offerings by amalgamating them with new technologies and new partners. This enables them to offer the entire end-to-end solution that no vendor today covers alone. For example, digital twin vendor Siemens teamed up with Nvidia in a partnership that supports both companies’ ecosystems.
- Digital twins are not always based on standalone solutions. Many digital twin solutions are integrated within the larger software landscape (e.g., CAD, CAE, and simulation). Although the reference architectures we analyzed as part of the research highlight the need for digital-twin-specific software, it can also reside within a different or within a broader software offering.
Methodology
As part of our new 233-page Digital Twin Market Report 2023–2027, we prepared a comprehensive database of 100 digital twin case studies with essential information regarding the particular companies, projects, and industries. The information was sourced through both primary and secondary research—conducting qualitative research at conferences such as the European Digital Twin Summit or Hannover Messe 2022, webinars, analyst briefings, and one-to-one meeting with industry experts.
The case studies were included in the report on the basis of sufficiency of information and satisfaction of the core requirements for being called a digital twin, as per IoT Analytics’ definition.
Are you interested in learning more about Digital Twin applications?
IoT Analytics is a leading global provider of market insights and strategic business intelligence for the Internet of Things (IoT), AI, Cloud, Edge, and Industry 4.0.
Digital Twin Market Report 2023-2027
A 233-page report detailing the market for digital twins, including definition & disambiguation, standardization efforts, market size & outlook, competitive landscape, market hotspots, case studies, trends & developments.
Click on the button to load the content from .
Related publications
You may be interested in the following publications:
- Enterprise Augmented/Mixed Reality Market Report 2022–2027
- Digital Supply Chain Market Report 2022-2027
- Industry 4.0 Adoption Report 2022
- Industrial Software Landscape 2022–2027
- Cloud Computing Market Report 2021–2026
Related articles
You may also be interested in the following recent articles:
- The enterprise AR market and the industrial metaverse: Why 2023 marks an inflection point
- 8 key technologies transforming the future of global supply chains
- The rise of Industry 4.0 in 5 stats
- The top 10 industrial software companies
- The case for a $2 trillion addressable public cloud market
Related market data
You may be interested in the following IoT market data products:
Global IoT Enterprise Spending Dashboard
Are you interested in continued IoT coverage and updates?
Subscribe to our newsletter and follow us on LinkedIn and Twitter to stay up-to-date on the latest trends shaping the IoT markets. For complete enterprise IoT coverage with access to all of IoT Analytics’ paid content & reports including dedicated analyst time check out Enterprise subscription.